Fine-Tuning LLMs With Retrieval Augmented Generation (RAG), by Cobus Greyling
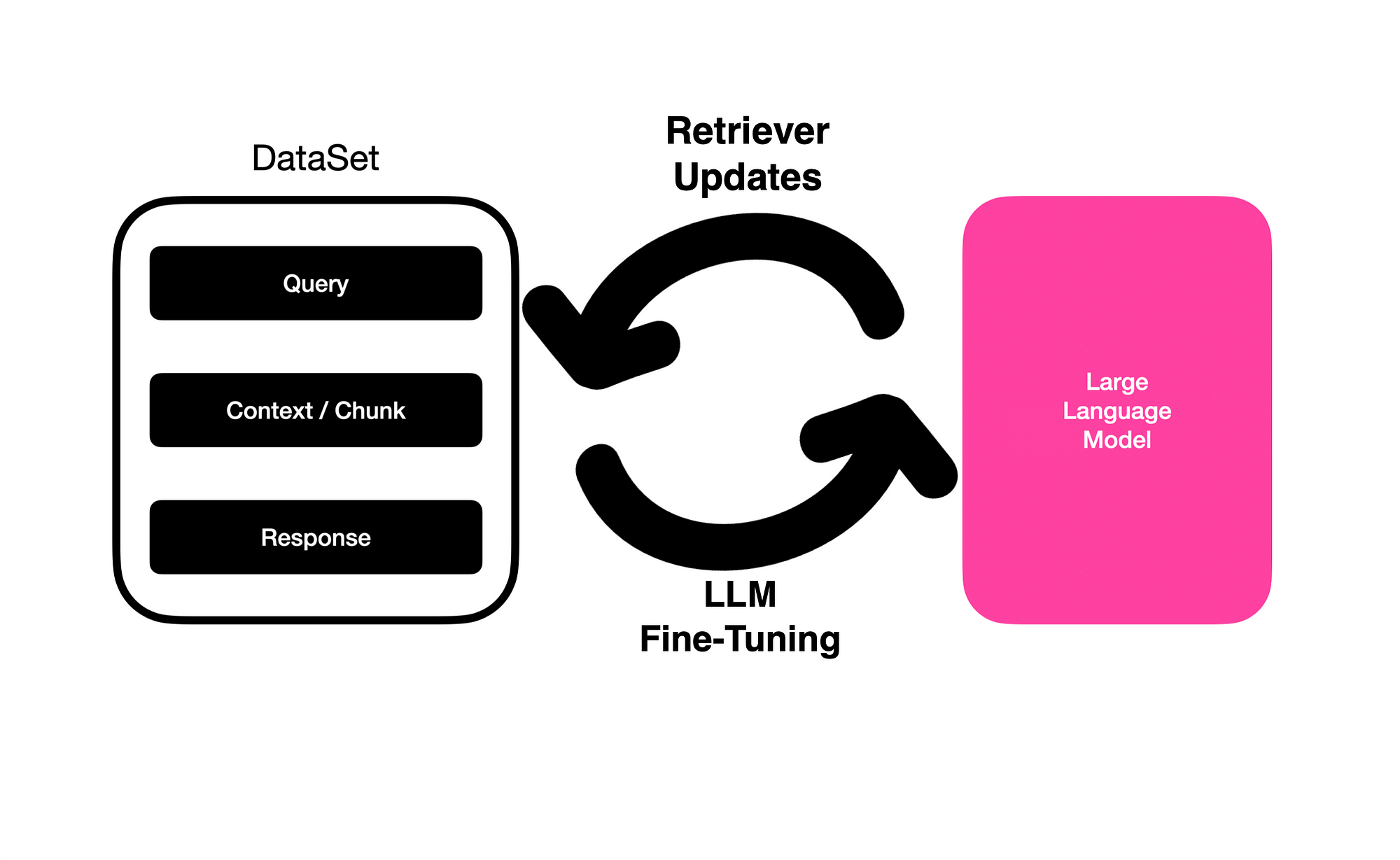
This approach is a novel implementation of RAG called RA-DIT (Retrieval Augmented Dual Instruction Tuning) where the RAG dataset (query, context retrieved and response) is used to to fine-tune a LLM…
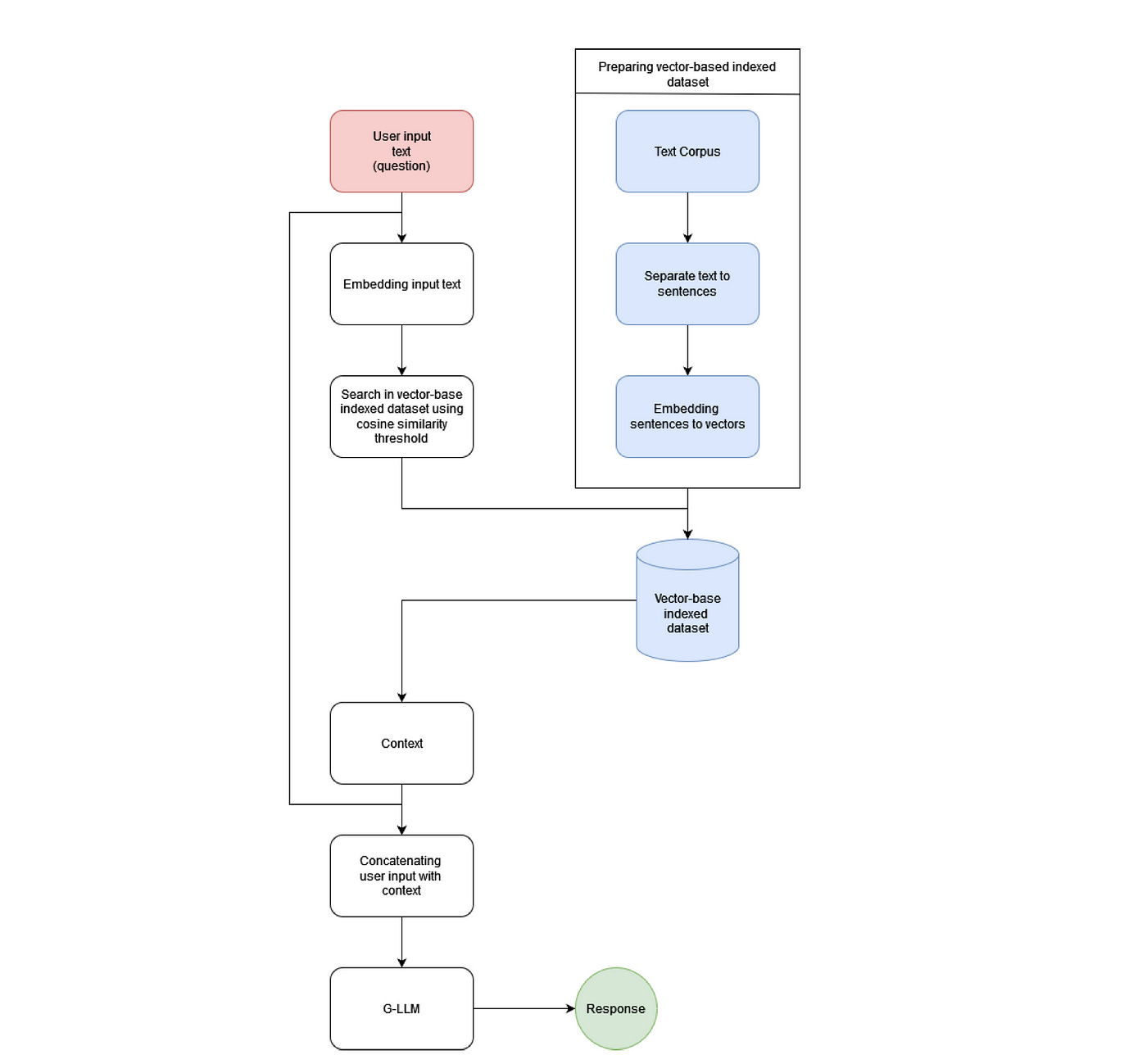
A New Study Compares RAG & Fine-Tuning For Knowledge Base Use-Cases
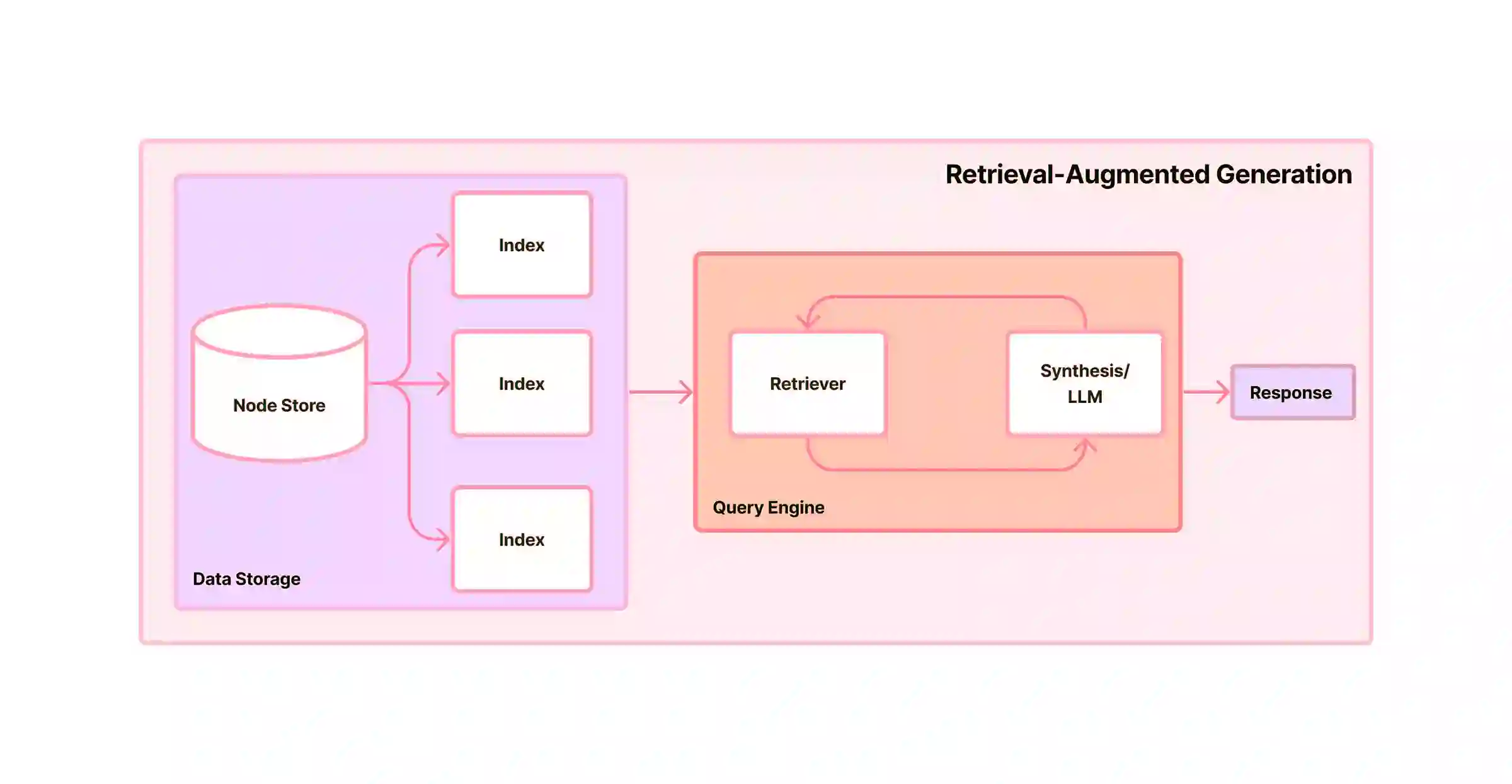
Retrieval Augmented Generation for LLM Bots with LangChain
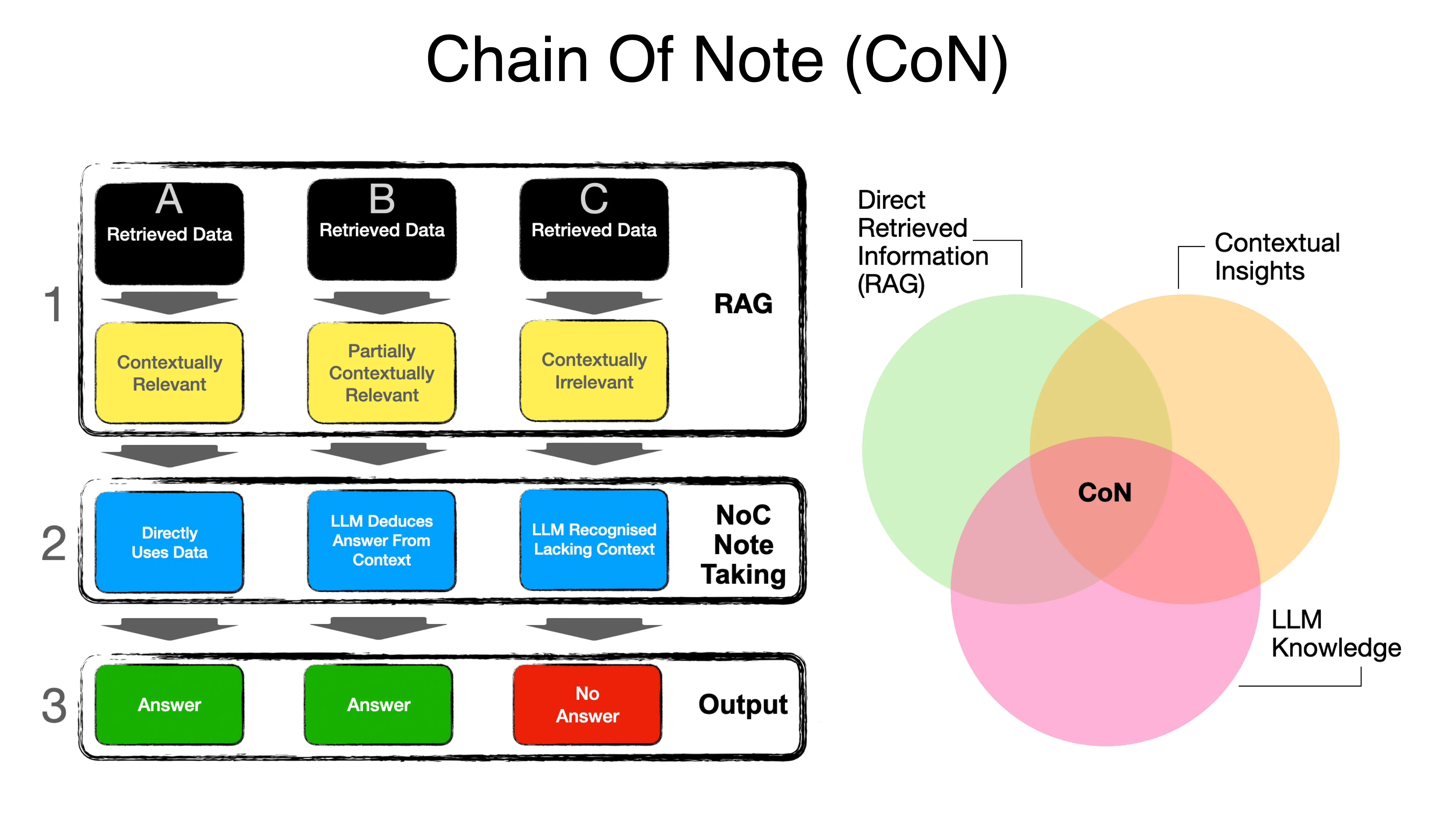
Chain-Of-Note (CoN) Retrieval For LLMs

Advanced RAG 01: Problems of Naive RAG
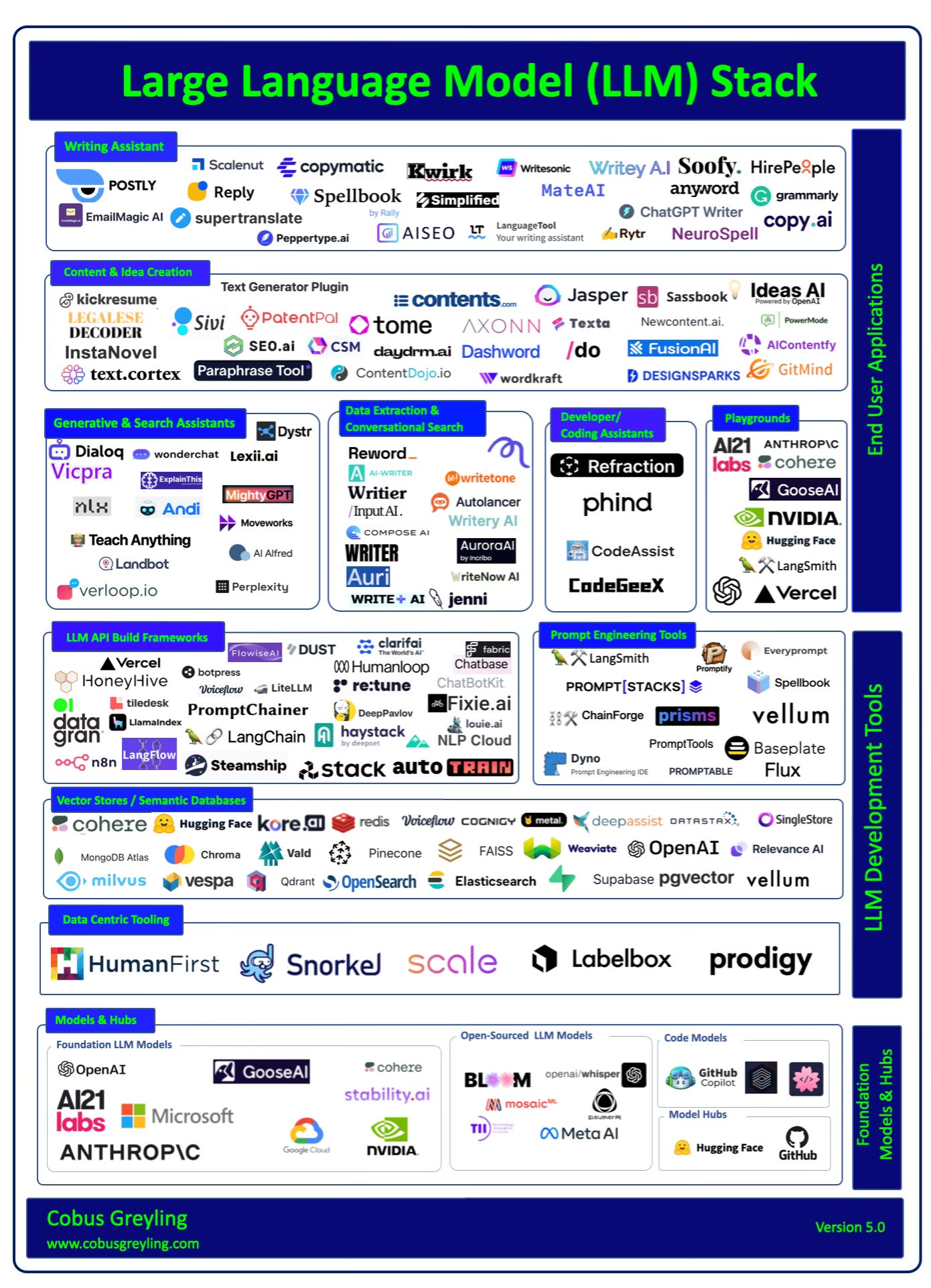
Large Language Model (LLM) Stack — Version 5
Cobus Greyling on LinkedIn: How To Fine-Tune A Large Language
Cobus Greyling on LinkedIn: Updated: Emerging RAG & Prompt
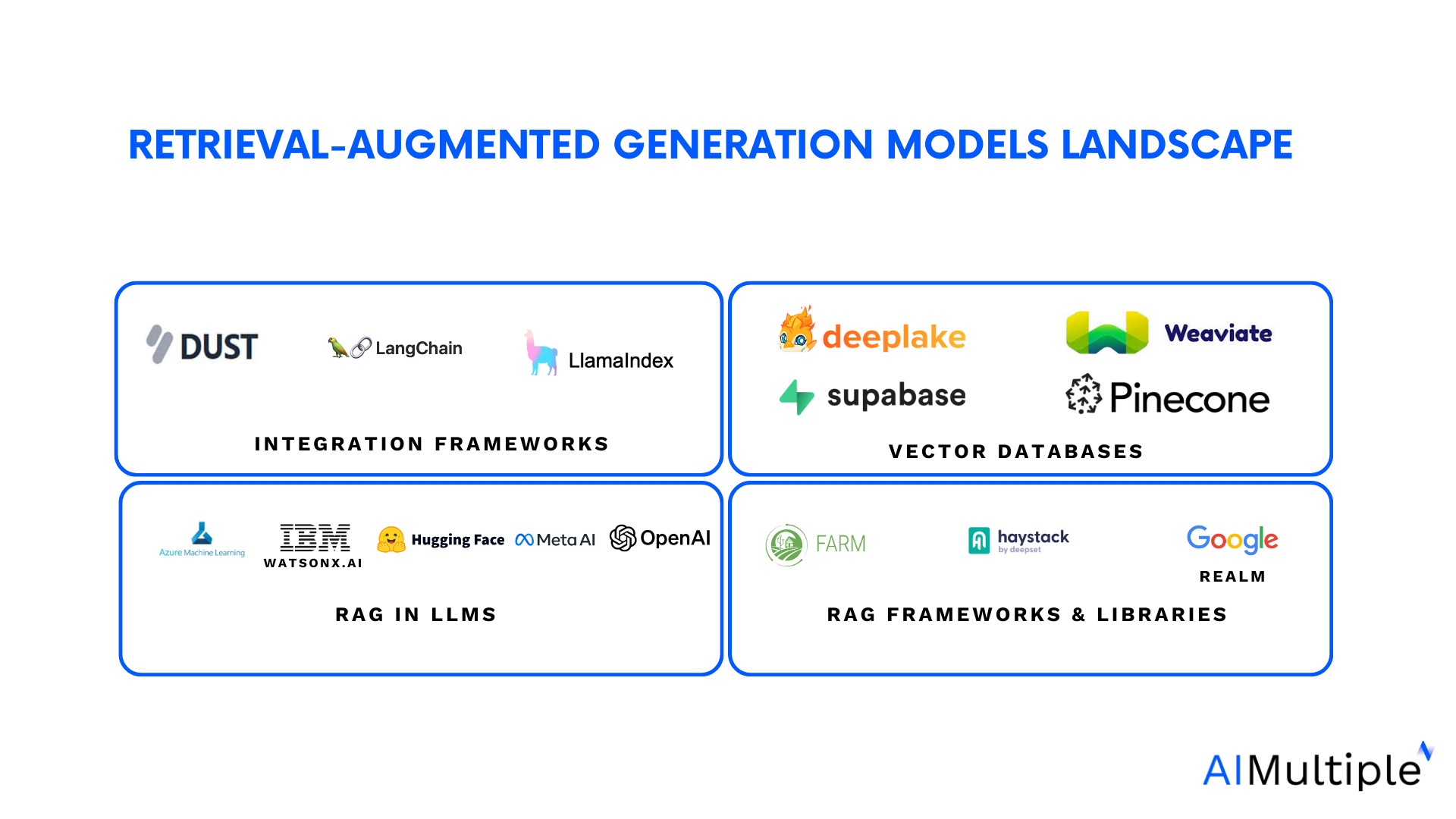
12 Retrieval Augmented Generation (RAG) Tools / Software in '23
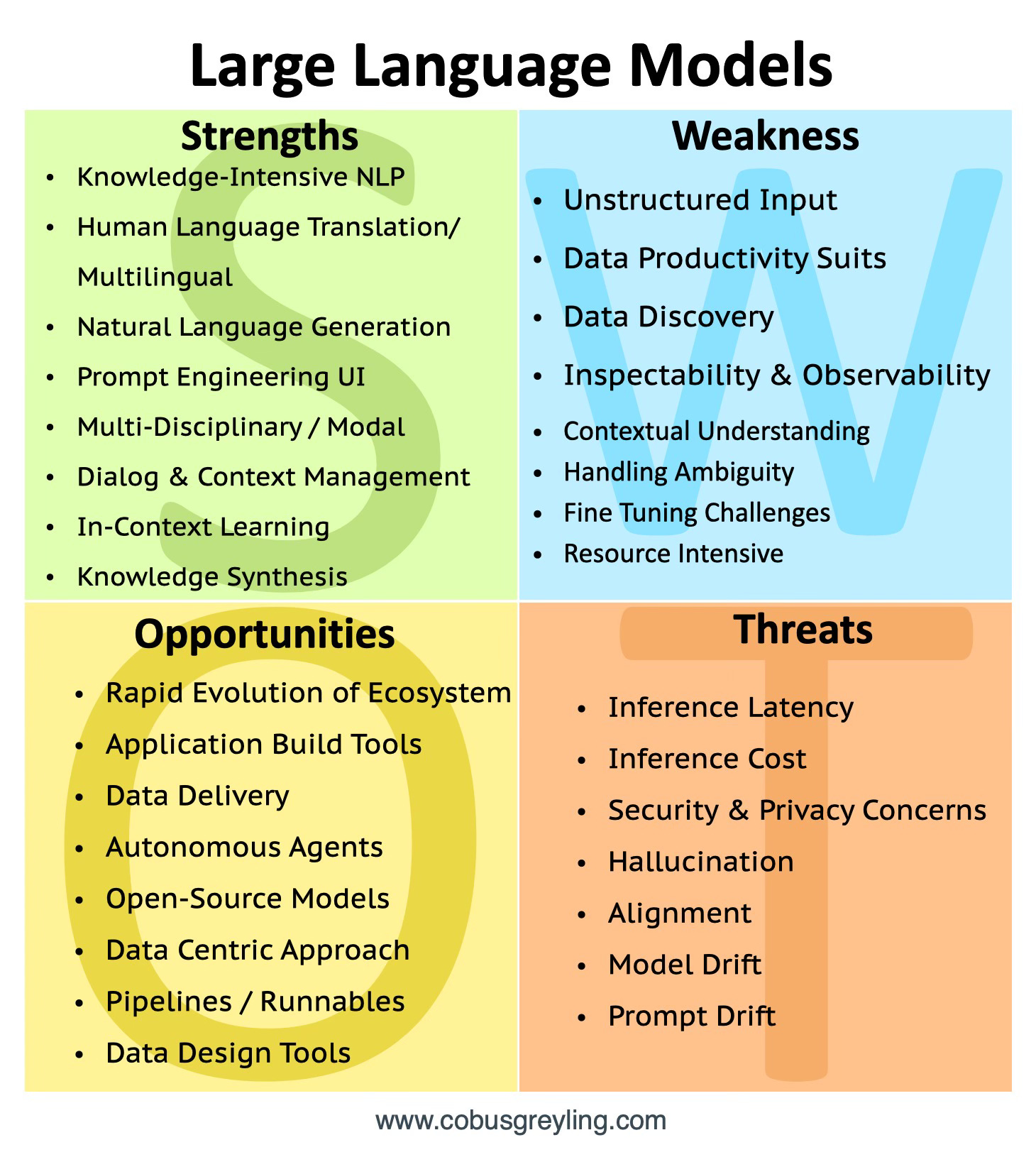
Large Language Model (LLM) SWOT Analysis (Updated)
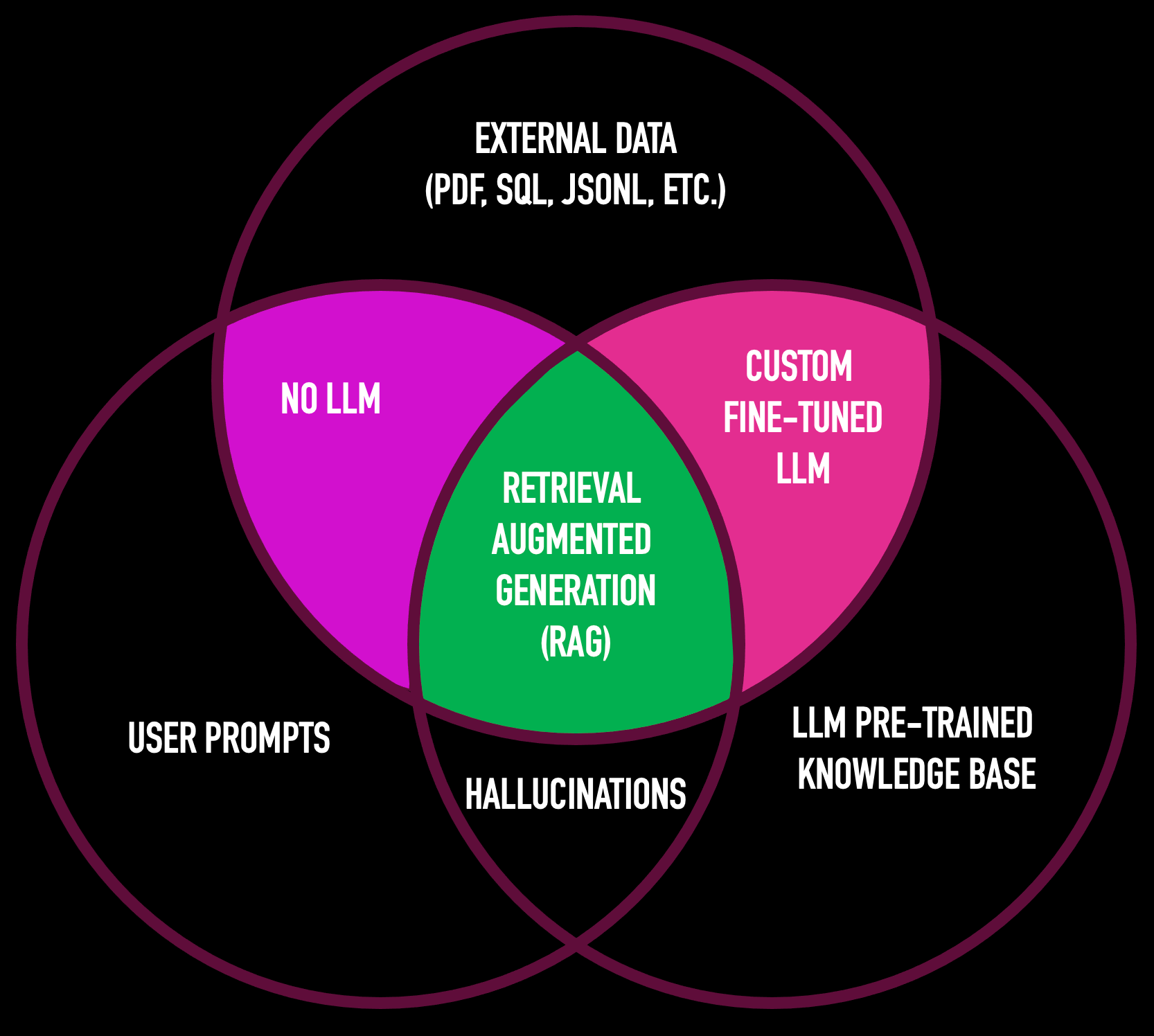
Fine-tuning an LLM vs. RAG: What's Best for Your Corporate Chatbot?
Cobus Greyling on LinkedIn: LLM Drift
Amit Sharma on LinkedIn: #generativeai #rag #finetuning
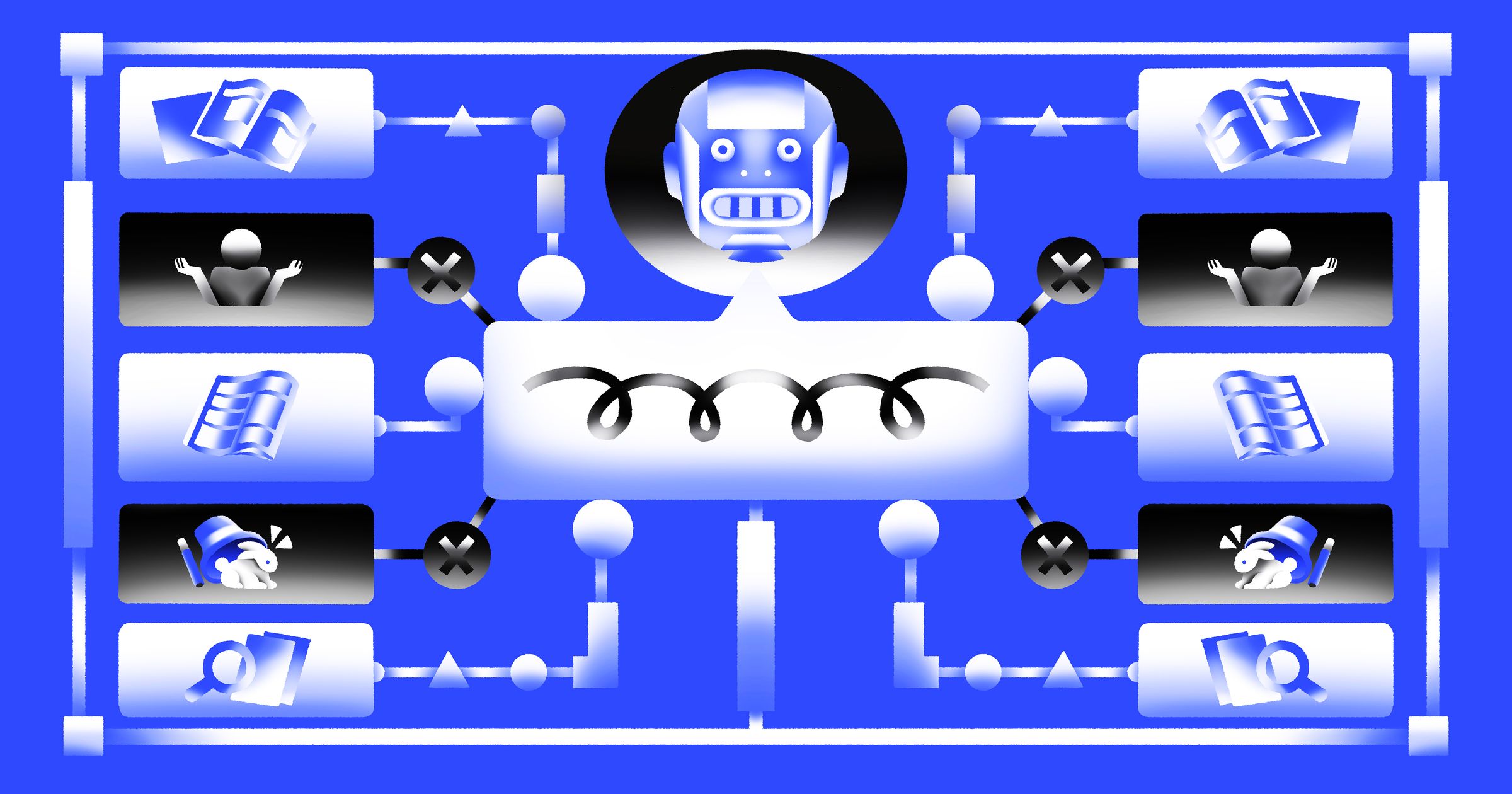
Retrieval augmented generation: Keeping LLMs relevant and current
Cobus Greyling on LinkedIn: Due to the highly unstructured nature